What the Heck is an Analytics Strategy? And Why Do You Need One?
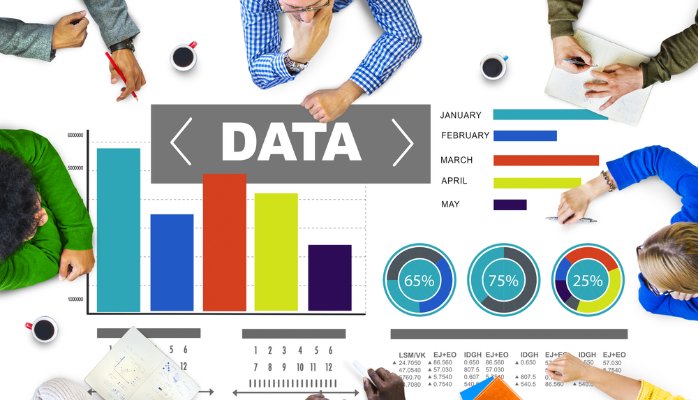
Many analytics projects flat out fail, others go over budget, over schedule and end up not delivering the promised business results. There are many reasons for these failures. Gartner listed 8 of them in a recent article: management resistance, selecting the wrong uses, asking the wrong questions, lacking the right skills,anticipated problems beyond big data technology, disagreement on enterprise strategy, big data silos and problem avoidance. The list can go on. The real question is not why these projects fail, but how you can avoid costly failures.
The key to success is s a clearly defined vision and a strategy. Whether you are planning an enterprise wide analytics program to transform the business or an analytics initiative that would benefit a single department, a well-crafted Analytics Strategy can make all the difference by proactively addressing the reasons for the failures.
A Program, Not a List of Point Solutions
The ultimate measure of the success of an analytics initiative is the resulting business benefits. To realize these benefits one has to apply the analytics to decision making, to improve business efficiency, to reduce costs, to go after new businesses and drive growth and create a competitive advantage. The new reality enabled by analytics is going to challenge established business processes and job responsibilities of many individuals. This is wholesale change.
There are two ways to approach this change, bottoms-up or top-down. In practice, many analytics initiatives take bottoms up approach, geared towards small changes that do not require a big strategy. This approach generally ends up with point solutions implemented in silos. In the best-case scenario, the business impact of such implementations are marginal as the implementation does not scale, duplicates data, not easy to maintain, not sustainable and have the many of the other issues Gartner has pointed out. In the worst-case scenario such implementations just fail or do not get integrated into decision making process, thus failing to deliver the business impact
With a Business Case and a Roadmap
The top down approach starts with the business impact and plan for addressing the change head on to transform the business to leverage the power of data driven decisions. This approach ends up with creating a program with a defined roadmap to roll out analytics capabilities to the businesses in a well-planned phased manner.
The key tothe success of this approach is to ask the right business questions up front, such as: Where in the business processes can analytics be applied? What are the quantifiable benefits of doing so? What does it take to launch a program to adopt analytics? How much and how long it is going to take? And what are the risks and how to mitigate them?
Business transformation is not an isolated activity. Analytics strategy in this regard has to be very closely aligned with the business strategy. Many times analytics is not one of the core competencies of the businesses considering adoption. This is where the well-crafted analytics strategy will identify where the gaps in terms of organization and people, processes, technology and data are and proactively address them to be successful.
A Foundation to Build On
It is also important to pay attention to the characteristics of a well-designed roadmap. It is not a set of projects that deliver a list of point solutions, as many do in practice. It is a carefully crafted list of phases that are designed to incrementally build the foundational capabilities that take advantage of low hanging fruit and deliver business impact early and often as the program is implemented.
This foundational capability needs to be conceptualized as a comprehensive analytics platform encompassing people, process, technology, data management, reporting and analytical capabilities that will mature through the phases of roadmap implementation.
An Organized Group of People
How you put together the right organization and resources with the required skill set has a very direct correlation to the success of the program. Hiring few data scientists and wait for the magic to happen may not be a good strategy.
Analytics is not pure business or technology. Each business is unique in their own way. Therefore, for many organizations a custom organizational model that interfaces with business functions and IT is required to ensure the development and adoption of analytics that results in business impact.
The required skills needed include; leadership, management, business, quantitative modeling and technology skills. There are many shapes and colors inside each of these skill categories. For many organization these skills are outside of their core competencies and hard to come by. Sometimes the required skill sets may exist internally, assuming they can be freed up from their existing responsibilities.
Established LEAN Processes
At a high level there are two sets of processes – the business processes that benefit from the application of analytics and the processes related to producing and supporting analytics. In the first set there often opportunities to redesign the process during the transformation from the current state to the analytics enabled future state that often gets missed. A good rule of thumb is to LEAN these processes before automation and application of analytics.
There are opportunities to leverage the existing business and IT process for the second set. New processes can be established to fill the gaps while improving existing processes.An audit of both sets of processes to identify their current state and then developing recommendations for process improvement is a necessary output of a good strategy engagement.
Well Managed Data Assets
Data management is one of the most important and at the same time a skilled labor-intensive task. This where the heavy lifting is required, in terms of cost and time.
Data management can be thought as similar to the several steps of value addition that take place during the processing of a diamond; from finding it in the rough to taking it to market.
Poorly managed data can impact the quality of analytics produced, productivity of the processes and ultimately the business impact, similar to poorly processed diamond will have very little street value. The data management steps include Data Design (Drawing/marking), Data Integration (Cleaving/sawing) Master Data Management, Data Quality (Bruiting) and Preparing Data for Analytics (Polishing). There are several possible variations to the steps depending on whether you are working with a traditional data warehouse, a more modern big data architecture or a hybrid environment.
Carefully Selected Set of Technologies
Jumping the gun and buying lot of technologies do not necessarily make your odds of success in analytics initiatives any better. Contrary to the common sense, this what exactly many businesses do, they go for the magic bullet.The answers lay in the technologies many of the companies already own, and identifying gaps and filling them with the complementary technologies. Technology landscape is not very easy to decipher, with lots of overlapping capabilities, options and constraints.
A well-designed strategy engagement can help pick the right set of technologies to build the comprehensive analytics platform taking advantage of the many options from many of the leading vendors and open source technologies. General characteristics of good technologies include: ease of implementation and support, high performance, ease and high productivity of development that reduces the time –to-value during the implementation. During an analytics implementation the cost of technology is relatively small portion of the total cost of ownership over a period of time. The higher cost components are associated with labor to develop and maintain. Therefore, paying more to acquire the right technologies is not necessarily a bad thing.
This post taken from http://ciostory.com
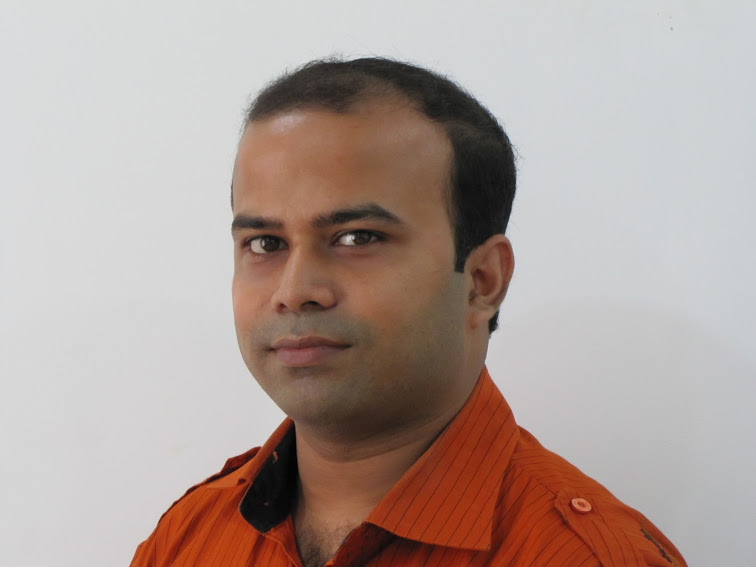
Kalyan Banga226 Posts
I am Kalyan Banga, a Post Graduate in Business Analytics from Indian Institute of Management (IIM) Calcutta, a premier management institute, ranked best B-School in Asia in FT Masters management global rankings. I have spent 14 years in field of Research & Analytics.
1 Comment
Nancy Anderson
February 12, 2020 at 7:53 amHey,
i glad to read it, thanks for sharing a nice blog with us. it is too important to me.