Big Data 2017: Future is cloudy
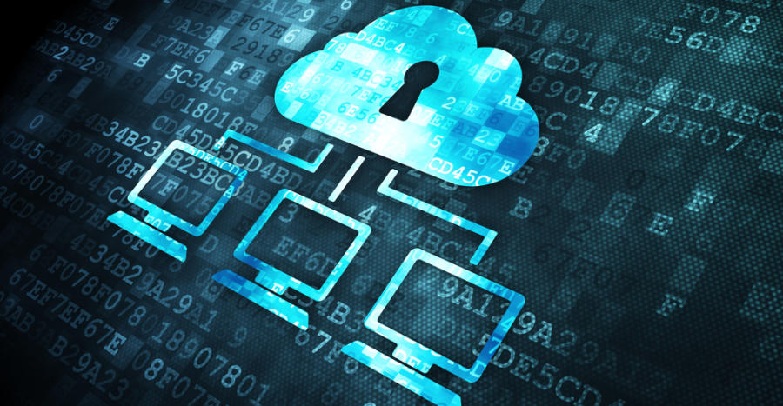
While machine learning and IoT grab the headlines, in 2017, cloud adoption will become the sleeper story of Big Data.
The old adage about two heads being better than one can well apply to this blog — except in this case, it’s three heads.
Last week, Andrew and George unveiled their crystal balls, and there was more than passing similarity to what we laid out in our Ovum forecast. For the record, we predicted that machine learning would be the biggest disruptor for Big Data analytics going forward. It’s hard to ignore the machine learning and AI juggernaut. If you buy products on Amazon or eBay, communicate with friends through Facebook, network through LinkedIn, or stream entertainment on Netflix, your experience is being shaped by machine learning models suggesting what products, promotions, friends, professional contacts, or videos might be most relevant to you.
The conundrum, as George wrote, is that “the masses are still trying to come to terms with Machine Learning.” As we learned, the key to success with machine learning and AI isn’t necessarily brain surgery. It’s about forming the right teams because the data scientist, no matter how brilliant or creative, cannot guarantee insights or discoveries alone; successful data science is a team sport.
As we noted several months back, data scientists may know their way around algorithms, but not necessarily how to get them to run on the cluster. That’s typically the role of data engineer, and according to conclusions reported by Andrew from DataStax, the term (and we presume, role) of data scientist might become subsumed by data engineers. We’d put a slightly different spin: data scientists won’t become less relevant, but demand for data engineers will keep outstripping them. It presages the need to get both on the same page, along with business analysts and subject matter (domain) experts.
And so we’ll continue to see more tools and frameworks for getting data scientists connected. Offerings like IBM Watson Data Platform, which provide integrated workspaces for each of the roles; Alpine Data Lab, which provides a collaboration environment for data scientists and business analysts; Dataiku, which offers an integrated analytics tool with connectors to data sources, visual data prep, and a choice of roughly 30 prepackaged ML algorithms; Domino Data Lab, which provides lifecycle management for ML projects; and Alteryx, which combines self-service analytics with a back end for developing ML programs. This is just the tip of the iceberg; in the new year, we’ll see more offerings that get data scientists and data engineers connected.
In Andrew’s piece, StreamSets CEO and founder Girish Pancha was quoted predicting that in 2017, “organizations would stop putting IoT on a pedestal.”
We believe that IoT is the use case that will push real time streaming onto the front burner. It’s the result of a perfect storm: open source lowered barriers to entry for what had been largely expensive, proprietary technology; commodity hardware made processing of large torrents of streaming data affordable and feasible; and bandwidth and the equivalent of Moore’s Law for sensors made smart devices more ubiquitous and increasingly connected.
But to avoid becoming a victim of its own success, IoT traffic must be managed. That explains, not only the vast growth of streaming analytics technologies like Spark Streaming, Storm, Flink, Apex, SQLstream, Kinesis, Heron and others, but also offerings that mediate data flows and queuing, such as Kafka, MapR Streams, Apache NiFi (productized by Hortonworks), Teradata Listener and others. It also raises the urgency of keeping chatty sensors from overwhelming the network.
And that explains why Amazon has extended outside its comfort zone with a client-side appliance, Greengrass, that provides some Lambda processing on premises to reduce and cache some of that IoT chatter before it gets to the Amazon cloud. Look for more such offerings to come in 2017, as growing embrace of IoT raises awareness for heroic measures to keep bandwidth from getting overwhelmed.
And increasingly, Big Data, whether from IoT or more traditional sources, is going to live and be processed in the cloud. This year we expect about 35 – 40% of new Big Data workloads to be cloud-bound; we expect that the inflection point — where the majority of new Big Data workloads are deployed in the cloud — will happen by no later than 2019.
While in his post, George posited that there would be limits – he equates cloud vs. on premises as a rent vs. buy decision, we believe other factors will increasingly make cloud deployment of Big Data the norm, and on premises the exception. There are the usual suspects such as the urge to shift costs from capital to operating budgets; speed of deployment; and data gravity. And with higher performance compute engines like Spark, the penalty of cloud-based architectures (where storage is separated from compute) will grow more trivial.
But we believe a couple factors that are especially relevant to Big Data will push the issue over the top. First, there’s the complexity of setting up Hadoop, a hurdle that impacts new adopters who lack the IT resources of the pioneers. But ultimately, the issue of security will be the clincher. As data lakes store more data — and with it, the likelihood that those data sets will contain highly sensitive data — the need to secure them grows more paramount compared to the early days when Hadoop only stored anonymized clickstream data. In an era of rapidly morphing exploits and hacks, who is better prepped to deal with attacks? Enterprise IT, or the cloud provider who makes infrastructure their core business?
Author: Tony Baer (Ovum) Source: ZDNet
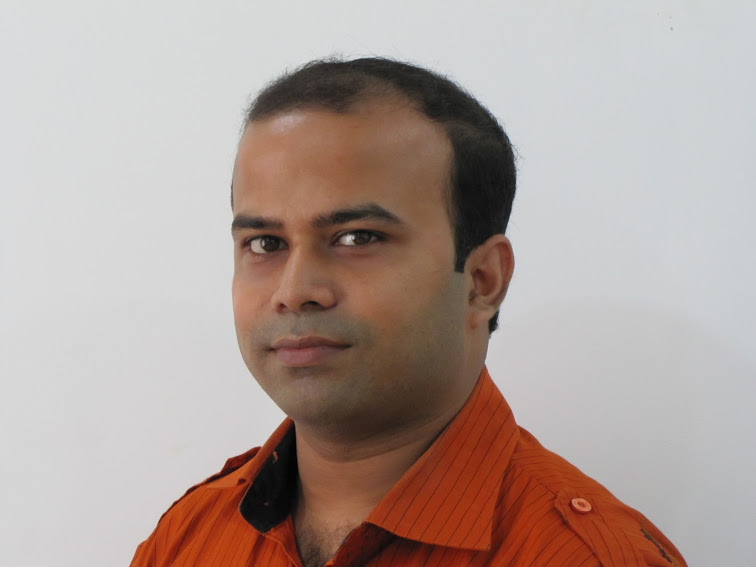
Kalyan Banga226 Posts
I am Kalyan Banga, a Post Graduate in Business Analytics from Indian Institute of Management (IIM) Calcutta, a premier management institute, ranked best B-School in Asia in FT Masters management global rankings. I have spent 14 years in field of Research & Analytics.
1 Comment
Speech Analytics Market to reach $1.6Bn by 2021 - Fusion Analytics World
April 16, 2018 at 2:02 am[…] Million in 2016 to USD 1.59 Billion by 2021, at a Compound Annual Growth Rate (CAGR) of 18 .0% . Cloud based deployment model will show a faster growth rate compared to […]